I’m an avid supporter of large-scale genomics studies, which have yielded some of the most important resources in human genetics and cancer genomics. With my new focus on inherited disorders that affect children, I have a new appreciation for initiatives that tackle not-so-common diseases.
One such effort is the UK-based Deciphering Developmental Disorders (DDD) study, which recruited 13,000 patients with undiagnosed developmental disorders from 2011 to 2015. Their penultimate findings, published earlier this year in Nature, have implicated 14 new candidate genes for DDs and provided some fascinating insights into their genetic underpinnings. Yet I’m also discussing this publication because it illustrates some important considerations for sequencing studies of genetic disorders.
The DDD study performed exome sequencing on 4,293 families, predominantly as classic trios of an affected individual and his or her parents. Most affecteds (81%) had been screened for large duplications and deletions, which is typically done by array and captures events measuring hundreds of thousands or millions of base pairs. The authors also collected the family history and detailed clinical information (e.g. growth measurements, developmental milestones, and HPO terms).
De Novo Mutation Calling
The authors placed a primary emphasis on de novo mutations (DNMs) present in the affected child but not his or her parents. Well-established de novo mutation rates in humans predict that newborns harbor, on average, 0.5 DNMs in coding sequences. The authors used DeNovoGear and identified ~1.95 coding DNMs per proband. They acknowledge that this is too high, but argue that they were aiming for sensitivity and can address the lower specificity by independent validation.

DNM Filtering Stringency (DDDS, Nature 2017)
Their filtering strategy for candidate DNMs appears technically sound. Using a set of 1,133 trios for which all candidate DNMs underwent validation (3730 sequencing), the authors chose a posterior probability threshold leaning towards sensitivity (see right). They also applied some common sense filters:
- Requiring that GATK call the variant in the child but neither parent
- Removing DNMs with strand bias (Fisher p<10^-3) in supporting reads
- Removing DNMs at multiallelic sites or with 1+ supporting reads in both parents
If we assume a conservative validation rate of 80%, that would yield about 1.5 DNMs per proband (a 3-fold enrichment over expectation). Yet this may be the true underlying biology: by enrolling DD patients with predominantly healthy parents, they’ve almost certainly enriched their cohort for individuals harboring coding DNMs.
DD Gene Discovery
Of the 4,293 individuals in this study, 23% had “probably pathogenic” missense or truncating DNMs within a clinically curated set of dominant DD genes. To systematically search for frequently mutated genes in DDs, the authors combined their 8,361 candidate DNMs with 4,224 DNMs (3,287 individuals) from 13 published sequencing studies. Some 93 genes achieved genome-wide significance, of which 80 had previous evidence of association with developmental disorders.
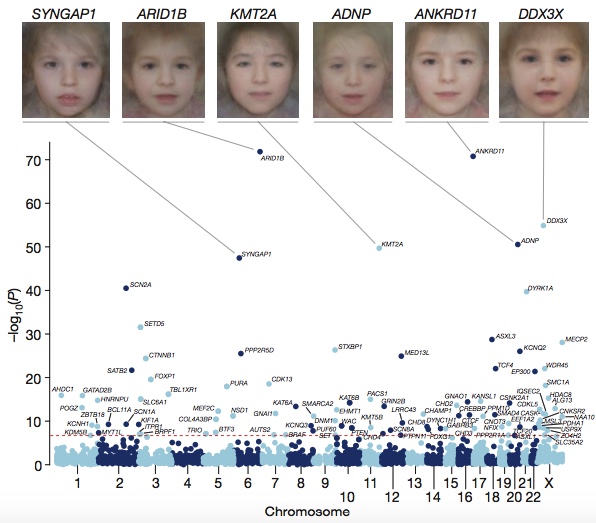
DD Associated Genes (DDDS, Nature 2017)
The authors performed some interesting analyses that I won’t cover in-depth here, such as:
- Creating composite face images from clinical photos of patients harboring mutations in the same gene to highlight common facial dysmorphology. It’s essentially the converse of the Face2Gene project. Honestly, the composites all look the same to my untrained eye; I find the example photos in Smith’s to be more useful.
- Integrating phenotypic data into disease gene association. Combining genetic evidence with HPO term similarity increase the significance for some genes, but decreased significance for a larger number of genes that are associated with DD and broader phenotypes.
About 20% of individuals in the cohort had clinical evidence of seizures and/or epilepsy, so the authors compared the analysis of this subgroup compared to the entire cohort for seizure-associated genes. Fifteen such genes achieved significance in both the whole cohort and the clinical seizure subset, but another 9 genes were significant only in the cohort analysis.
Furthermore, of the 285 individuals with pathogenic DNMs in seizure-associated genes, only about half (56%) had clinical evidence of seizures. The authors conclude that a large sample size easily outweighs phenotypic similarity for achieving statistical power to discover new disease genes.
Exome or Genome Sequencing?
The authors felt that the large number of significant genes provided an opportunity to compare exome and genome sequencing, with respect to their power to identify genes associated at genome-wide significance. They laid out the following assumptions:
- Genome sequencing costs $1,000 per sample.
- Exome sequencing costs 30-40% of that cost per sample
- Genome sequencing uncovers 5% more coding variants
- Project budgets is $1 million, $2 million, or $3 million
Under these assumptions, the authors found that “exome sequencing detects more than twice as many genome-wide significant genes.” Well, sure: if you can sequence 3 times as many samples with 95% sensitivity, you obviously have far more statistical power. Sample numbers always trump slight increases in variant detection sensitivity.
But let’s not forget that this is a gene-centric analysis focused on coding DNMs, which are accessible to both exome and WGS strategies. It ignores the classes of pathogenic variants that are refractory to detection by exome sequencing, such as noncoding and structural variants. These are harder to find and to distinguish from benign variation than coding variants. But if we don’t look for them, we’ll never fully understand the genetic architecture of inherited diseases.
Previous Testing and the Falling Hit Rate
The authors also noted that, although their power calculations estimated they’d identify about half of all haploinsufficient DD-associate genes at genome-wide significance. Empirically, they identified slightly less than half (47%). They hypothesized that a priori genetic testing may have depleted the cohort of easy-to-diagnose disorders. Indeed, an assessment of the DNMs in the most clinically recognizable DD genes showed a three-fold lower enrichment for truncating mutations than other DD genes. When these disorders were removed, they identified 55% of all haploinsufficient genes. Right on the money.
This example illustrates an idea about the feedback loop between clinical diagnostic sequencing and research discovery sequencing. In the first several years after exome sequencing was applied to rare disease diagnosis in appreciable numbers, researchers gradually came to recognize that the industry-wide “hit rate” — the proportion of sequenced cases achieving a molecular diagnosis — is typically 25-35% across centers and studies. Clinical and diagnostic laboratories have often adopted this as an informal benchmark for the success of their pipelines.
As this study illustrates, the proportion of cases that achieve a molecular diagnosis depends considerably on the extent of genetic (and biochemical) testing already performed. For completely naive (untested) individuals, we expect that hit rate to increase over time as new assays, methods, and knowledge improves our ability to find the underlying cause of disease.
Yet the improvement and widespread adoption of genetic testing means that such naive samples are increasingly rare. Most patients with a rare disease, particularly a recognizable one, will undergo a barrage of gene or panel testing (and often a copy number array) as part of the diagnostic process. As we find and establish new disease genes, that barrage becomes ever more effective at providing diagnoses before exome or genome sequencing are necessary.
In summary, we should not be alarmed (or self-critical) if the “hit rate” of exome and genome sequencing studies decreases over time. That is the expected outcome. In settings like ours, where research aims to inform clinical care, it’s actually a sign that we’re moving in the right direction.